Travel demand modeling is one of the most powerful tools for planners considering updates to their networks — but also one of the least accessible. Existing software for demand modeling is expensive, and often requires specialized training and staff. It’s something agencies only commit to — if they undertake it at all — as part of a major network redesign, rather than something they can play with as they test out ideas for more frequent network adjustments and optimizations.
And after all that, up-to-date origin-destination data is scarce and expensive, so many agencies rely on household travel surveys that quickly become stale. Some agencies we’ve spoken with even complain that their data is pre-Covid, and reflects drastically different demand patterns than currently in play.
A better solution? Meet Remix Ridership Modeling: an easy-to-use, lightweight demand prediction tool right within the familiar Remix interface. Remix’s planning tools will draw on an unprecedentedly-detailed — and continually-updating — set of origin-destination data from Citymapper to model how riders will behave when you make changes to your network.
What does Ridership Modeling let you do? As you’re planning a bus route in Remix, you can compare different options and scenarios to understand:
- How many riders currently take this route during the AM peak — and how might that change if we adjust the frequency?
- How long does it take riders to travel from a new housing development to a new job center — and how would adding a new bus route impact that travel time?
- Can we reduce riders’ walking time to transit by relocating stops closer to trip origins? How many minutes could we save?
Planners can examine stop-level boardings and alightings, and filter data by day of week and time of day.
With Ridership Modeling, travel demand modeling is an easy extension of your day-to-day work in Remix. You don’t need to worry about importing datasets, validating GTFS, or configuring a model — just make a network change, and click a button.
Want to skip ahead and see how a planner might use Ridership Modeling to answer questions about their network? Click here.
How it works.
There are three major components to Ridership Modeling in Remix, each of which answers a major question about your network:
- Where do people travel?
Detailed, up-to-date origin-destination data, collected and aggregated from anonymized Citymapper users planning trips in the app. - What are the best journeys?
A routing algorithm that plans trips across your existing and updated networks. - What would they choose?
A rider behavior model, trained on the real-life decisions Citymapper users make between the route options offered in the app.
How do these all work together?
- When you input your new transit scenario into Remix, Ridership Modeling gathers anonymized and aggregated information about riders who travel your network; where they’re coming from, where they’re going, and how they’re getting there now.
- Unlike the data supplied by household travel surveys, Citymapper data has real-time, ever-updating visibility into passengers’ origins and destinations, as well as travel times.
- Ridership Modeling then runs a routing algorithm across your new scenario, generating a set of journey options that your riders would encounter.
- Just like in Citymapper, these journey options include buses, trains, taxis, scooters — as well as just plain walking.
- Then, the rider behavior model makes the final “decision” for each rider in your analysis, choosing one of the options generated by the routing algorithm.
- Citymapper not only presents journey options to riders — it knows what they pick, and continually tailors journey proposals to reflect what riders are most likely to choose. This same information trains Remix's rider behavior model. And the model isn’t one-size-fits-all: it varies by city, just as rider preferences do.
- With journeys in your new scenario “chosen,” Ridership Modeling can estimate total ridership, as well as average wait times, walk times, total travel time and in-vehicle travel times. It then generates a comparison against the baseline scenario, highlighting the impact of your proposed network changes.
Ridership Modeling in action.
Let’s say you’re a transit planner in Springfield, a fictional mid-sized city. Public transit is strong in downtown Springfield, where Citymapper helps residents navigate a mix of bus and light rail, but less prevalent elsewhere in the city.
Over the past few years, Springfield has seen significant job growth in an outlying neighborhood, Waterville. These new jobs have attracted primarily younger workers who prefer to live downtown — but transit connections to Waterville are limited, and these young workers generally don’t own cars.
Though you think the solution might be to extend an existing bus line, Route 10, to Waterville, you have some questions:
- What are the predicted changes in ridership for Route 10? Will this impact wait times for passengers currently using Route 10?
- Will the anticipated ridership growth justify the cost of a new or extended bus route?
- How will a change in the network near Waterville impact the rest of your network?
- Will ridership on other routes be impacted?
- Will travel or wait times increase in areas you didn’t expect?
Here’s how Remix’s Ridership Modeling gives you a fast, inexpensive, and accurate way to navigate these questions and test your hypotheses.
Build your new network scenario using the familiar Remix interface.
You pull up your transit map in Remix. You draw an extension of Route 10 to Waterville, adding a stop along the way and a terminal near the new employment center, all within Remix with ease.
Use Ridership Modeling to validate your proposed ideas.
For a city of Springfield’s size, it takes about 15 minutes and runs in the background while you take a quick break.
Dive into your results at the network level...
You learn that extending Route 10 will have the following effects on your network as a whole:
- Total ridership is estimated to increase by 11% systemwide
- Average travel times increase slightly, as do wait times
- Walk times decrease slightly, and transfer times increase slightly
...The route and stop level...
You dig into route-by-route results, using Ridership Modeling's filtering feature. For Route 10 itself, ridership is expected to double, with your new terminal doing a brisk business. A few other routes that connect to Route 10 can also expect increased ridership, as travelers from Watertown connect elsewhere in the city.
... and by day and time.
You finally want to check your assumption that these ridership patterns are indeed driven by commuters working in the new job centers in Watertown. So you filter the results by day of week and time of day, and see that the network ridership increase is driven by weekday travelers in the mornings and evenings.
Easily visualize your results for key stakeholders.
You screenshot your proposed extension and its expected results, and plan to bring them to your next planning meeting.
Try it out.
Curious to see Ridership Modeling in action? Reach out; we'd love to talk.
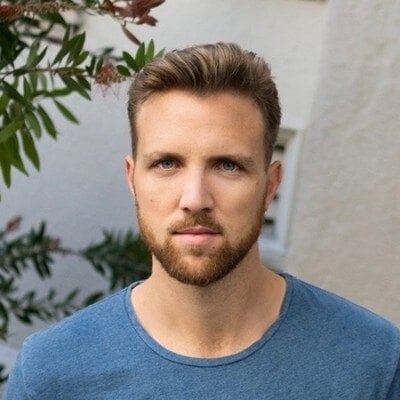
David is a Director of Product Management for Remix at Via.